A brand new period the place machine studying meets security in navigating complicated city terrains, promising a future the place self-driving vehicles adapt to evolving environments with out compromising security.
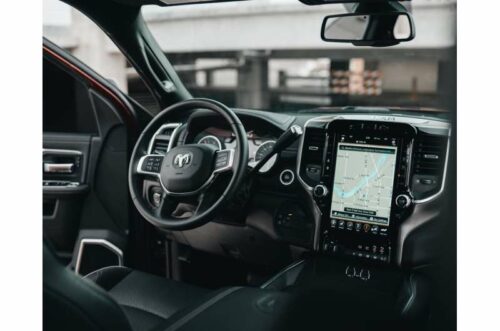
Over current years, synthetic intelligence (AI) and machine studying have been instrumental in steering self-driving vehicles in direction of superior operational capabilities. These vehicles are actually outfitted to analyse in depth knowledge via sensors, comprehending intricate environments and complying with visitors norms successfully. Nevertheless, when transitioning from managed environments to chaotic real-world streets, these automobiles usually encounter efficiency disruptions, heightening the chance of accidents.
In a stride ahead within the realm of autonomous car know-how, the NYU Tandon College of Engineering lately revealed an algorithm named Neurosymbolic Meta-Reinforcement Lookahead Studying (NUMERLA), crafted to surmount the enduring difficulty of unpredictability and security in real-world situations. Spearheaded by Quanyan Zhu, an affiliate professor {of electrical} and pc engineering, alongside his doctoral scholar Haozhe Lei, the innovation is poised to revolutionise how self-driving vehicles adapt and performance amidst the complexities of real-world visitors.
Taking a stride in bridging this hole between adaptability and security, NUMERLA emerges as a beacon of hope. This algorithm leverages the ability of real-time security constraint updates, empowering self-driving automobiles to traverse unanticipated situations whereas firmly upholding security protocols. Inside its framework, a self-driving automobile, upon encountering a altering setting, fine-tunes its understanding of the present scenario via collected observations. Consequently, it foresees its forthcoming efficiency over a predetermined interval, establishing appropriate security constraints and rejuvenating its information repository. This method primarily hinges on lookahead optimisation fused with security parameters, culminating in an empirically safe, albeit suboptimal, on-line management technique. Utilizing this algorithm, the car adjusts to new circumstances expeditiously with out compromising security.
Proof of the algorithm’s efficacy was witnessed throughout a simulation inside a digital platform mimicking city setups, the place its prowess in managing unpredictable situations like jaywalkers was distinctly superior to different present algorithms.